Explore the most popular computer vision applications for your industry. From retail to manufacturing to medicine, most companies have already started computer vision projects to increase productivity and accuracy within their respective fields. Let’s examine some of the best applications of computer vision in several industries.
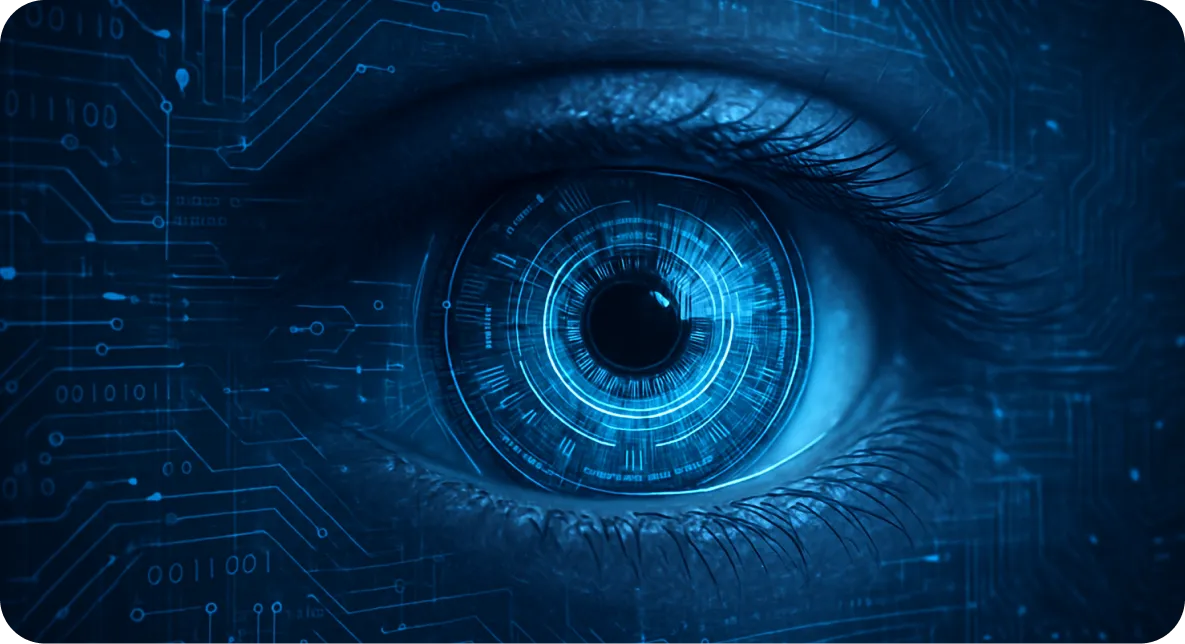
What Is Computer Vision?
Computer vision is a branch of artificial intelligence (AI) that leverages machine learning (ML) and deep neural networks (DNNs) to give computers the ability to perceive, recognize patterns, and interpret elements within digital images and video data in a way that is similar to human vision. This area, often referred to as computational vision, enjoys widespread adoption for applications like automated AI visual inspections, distant surveillance, and process automation.
Computer vision programs utilize artificial intelligence to allow computer systems to extract valuable insights from visual stimuli like image data and videos. The information derived from computer vision is later used to initiate automated responses. Just as AI equips computers with the capacity to reason, computer vision grants them the capability to perceive.
As humans, we spend our lives observing our environment through optic nerves, retinas, and the visual cortex. This process gives us the contextual awareness to distinguish between various items, estimate their proximity to ourselves and others, determine their velocity, and identify anomalies. Similarly, computer vision gives AI systems the power to teach themselves how to perform these same functions. These systems rely on a blend of camera sensors, mathematical models, and datasets.
However, unlike people, machines do not suffer from fatigue. Devices powered by computer vision can inspect thousands of components or goods within minutes. This innovation permits manufacturing facilities to automate flaw detection, including defects imperceptible to the human eye.
Computer vision works best with an extensive dataset because computer vision systems repeatedly evaluate training data until they extract every relevant detail necessary for their specific assignment. For example, a computer taught to identify healthy livestock must be exposed to tens of thousands of visual examples, including cows, sheep, wolves, fields, and other relevant elements. Only then can it accurately recognize healthy livestock types, distinguish them from unhealthy ones, assess diseases, spot pests, and detect predators in the vicinity.
Two core technologies power computer vision: convolutional neural networks (CNNs) and deep learning, a subset of machine learning.
Machine learning uses algorithmic frameworks to help computers interpret context through the analysis of visual data. When fed sufficient information, the model develops the ability to understand patterns and differentiate between visual inputs. Instead of receiving explicit programming to identify and separate images, the system leverages AI to learn independently.
Convolutional neural networks (CNNs) assist ML algorithms in perceiving images by dividing them into individual pixels. Each pixel is assigned a descriptor or classification. These labels are then processed using convolution operations, which involve merging mathematical functions to produce a third function. This approach allows CNNs to interpret visual data.
To simulate human visual recognition, neural networks carry out convolutions and assess the precision of their outputs through many iterations. Much like humans identify objects at a distance, a CNN begins by spotting basic forms and sharp contours. From there, it fills in missing details and refines its output through multiple passes until the system can confidently predict what it is analyzing.
Whereas CNNs handle static images, recurrent neural networks (RNNs) process video sequences, enabling computers to learn how successive frames are interconnected.
What Are Computer Vision Systems?
Computer vision systems utilize imaging devices to capture visual information, machine learning algorithms to analyze and interpret the visuals, and rule-based logic to facilitate automated, task-specific operations. Edge computing augments this process by enabling scalable, efficient, resilient, protected, and privacy-preserving deployments of computer vision solutions.
At AiFA Labs, we offer a comprehensive, all-in-one, advanced computer vision infrastructure: the Edge Vision AI Platform. This platform permits leading enterprises to build, launch, expand, and protect their computer vision systems from a unified environment.
Why Is Computer Vision Important?
Computer vision is important because it reduces manual labor and reduces costs. Although businesses have enjoyed access to visual data interpretation technology for a while, much of the workflow previously relied on time-consuming, error-prone manual effort.
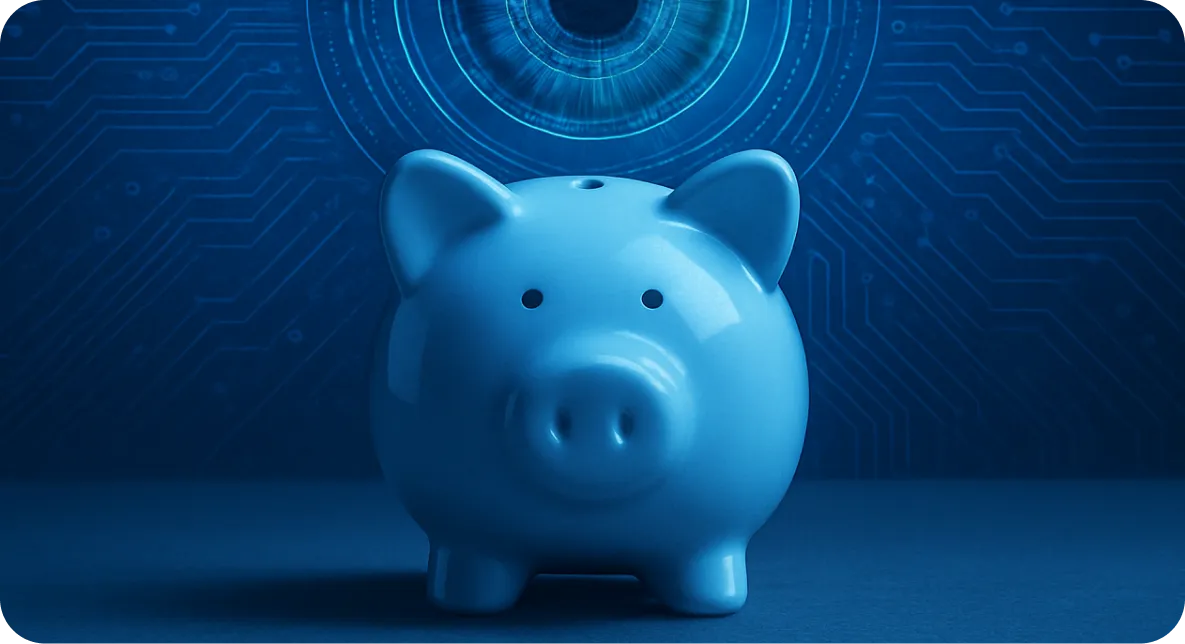
For instance, deploying a facial recognition system once required engineers to hand-label thousands of pictures with critical reference points, such as the width of the nasal bridge and the spacing between the eyes. Automating such processes demanded significant computational resources, as image-based content is unstructured and challenging for machines to categorize. As a result, vision-based applications proved costly and largely out of reach for many businesses.
Today, advancements in the technology, including a major boost in processing capabilities, have improved the scalability and precision of image analysis. Computer vision systems, supported by cloud-based infrastructure, are now widely available. Organizations of all sizes can leverage this technology for identity authentication, content filtering, real-time video interpretation, defect detection, and a range of other use cases.
Computer Vision Applications by Industry
Although the capabilities of the human eyes perform well, present-day computer vision looks primed to catch up. Some computer vision tasks are so complex that humans could never even dream of completing them. Below, find the top applications for computer vision of today.

Computer Vision Applications in Retail
Surveillance devices in retail environments permit store owners to gather visual information to create more optimized shopping and staff experiences.
The advancements in computer vision technologies’ ability to interpret this data have made the digital modernization of the physical retail sector significantly more achievable.
This innovation has recently gained support from improvements in receipt interpretation through generative AI (GenAI).
Find some of the most widely used retail computer vision use cases below.

Automatic Inventory
Computer vision platforms acquire visual data and conduct a comprehensive inventory check by monitoring products on shelves every millisecond.
The system can deliver real-time alerts about out-of-stock items and recent transactions, assisting personnel with stock control and inventory oversight.
Customer Tracking
Deep learning models process live video feeds to examine customer traffic within retail environments. Camera techniques unlock the repurposing of footage from standard, low-cost security cameras. Machine learning systems identify individuals in a non-intrusive, privacy-preserving manner to evaluate time spent in store sections, waiting durations, queue lengths, and service performance.
Customer behavior insights help managers optimize store layouts, increase shopper satisfaction, and track key performance indicators.
Occupancy Counting
Computer vision algorithms train on labeled datasets to identify individual items and tally them instantly. Human counting solutions assist retailers in gathering insights about their store performance. During the COVID-19 outbreak, this technology monitored customer presence when authorities enforced occupancy limits in physical stores.
Self-Checkout
Self-service checkout is better than ever due to computer vision-powered systems that interpret customer actions and track item movement.
Many stores have adopted cashier-free payment solutions to address retail challenges like lengthy lines.
Social Distancing Compliance
To guarantee compliance with safety protocols, organizations deploy proximity detection tools. A camera monitors the movements of staff and customers, utilizing depth-sensing technology to evaluate the spacing between individuals. Based on their relative location, the system displays either a red or green indicator around a person.
Theft Detection
Store operators can identify unusual activities, such as lingering or entering restricted zones, through computer vision algorithms that independently evaluate the environment.

Wait-Time Analysis
To avoid frustrated shoppers and lengthy queues, merchants deploy line monitoring systems. Queue detection leverages multiple cameras to observe and tally the number of customers in a line. When the number of people exceeds a predefined limit, the system triggers a notification for staff to activate additional checkout counters.
Computer Vision in Manufacturing
The manufacturing sector has already embraced several automation technologies, with computer vision serving as a core component.
It supports the automation of quality assurance, reduces hazard-related risks, and enhances operational productivity.
Find some of the most prevalent uses of computer vision in manufacturing processes, below.
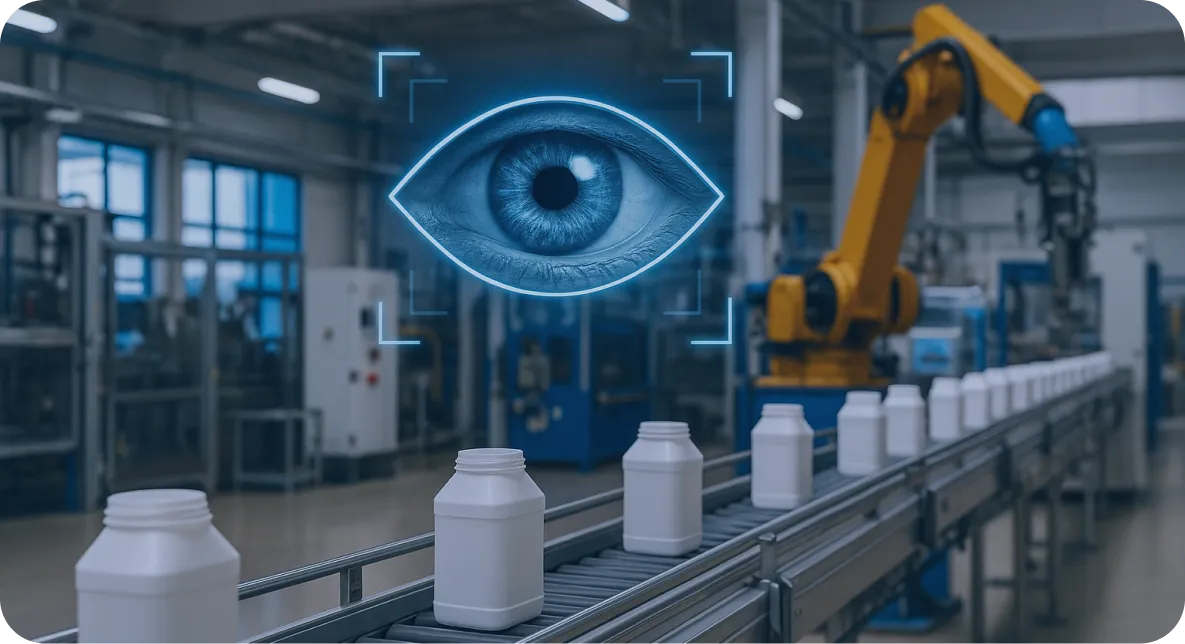
Employee Monitoring and Training
Another application for vision technologies involves the optimization of assembly line workflows in industrial manufacturing through human-robot collaboration. Analyzing human behavior assists with building standardized action templates linked to various manufacturing processes and assessing the effectiveness of trained personnel.
Automatically evaluating the quality of worker movements offers advantages like boosting job performance, increasing production efficiency, and identifying hazardous behaviors to reduce the risk of workplace accidents.
Equipment Inspections
Computer vision for image-based inspection is a crucial approach in intelligent manufacturing. Vision-based evaluation systems automate the assessment of personal protective equipment (PPE), including mask recognition and helmet identification. Machine vision supports compliance with safety regulations in construction zones and digitally advanced production facilities.
Label Verification
Since the majority of products feature barcodes on their packaging, many companies use a computer vision method known as optical character recognition (OCR) to automatically detect, validate, convert, and translate barcodes into readable text.
By using OCR on captured images of labels and packages, the system extracts embedded textual content and cross-checks it with existing databases.
This process assists in identifying incorrectly tagged goods, providing details about expiration timelines, reporting on inventory quantities in storage, and tracking shipments at every phase of the product lifecycle.
Product Assembly
Industry-leading enterprises like General Motors have already adopted automated systems for their product assembly operations. The company disclosed that more than 50% of its production workflows have been automated.
Computer vision enables the creation of three-dimensional design models, assists robotic systems and human operators, detects and monitors individual product parts, and supports the enforcement of packaging quality standards.
Productivity Analysis
Efficiency analytics monitor the effects of workplace adjustments, how staff utilize their time and assets, and the adoption of different technological tools. This type of data offers valuable intelligence on time utilization, teamwork dynamics, and worker performance. Computer vision-based, lean management approaches strive to objectively measure and evaluate workflows using camera-backed vision systems.
Quality Control
Intelligent camera solutions offer a scalable approach for deploying automated visual inspections and quality assurance in production workflows and assembly lines in smart manufacturing facilities. In this context, deep learning techniques apply real-time object recognition to deliver improved precision, processing speed, impartiality, and consistency, outperforming time-consuming manual inspections.
In contrast to conventional machine vision systems, AI-powered visual inspection employs highly resilient machine learning algorithms that depend on costly, specialized cameras or rigid configurations. As a result, these AI vision techniques prove highly adaptable and easily deployed within multiple manufacturing facilities.
Computer Vision in Agriculture
Agriculture is receiving a technological upgrade through the integration of computer vision solutions in the farming sector. Intelligent farming systems leverage visual information and ML algorithms to optimize current agricultural methods and operations. Discover some of the best examples of computer vision in agriculture, below.

Automated Farms
Technologies like harvesting, planting, and weed-removal robots, self-driving tractors, vision-based systems for overseeing distant farmland, and drones for aerial visual assessment boost efficiency even during workforce shortages. Profit margins increase by automating manual inspections using AI-powered vision, minimizing the environmental impact, and refining decision-making procedures.
Automated Harvesting
In conventional farming, mechanized tasks have long depended on manual crop collection, leading to elevated expenses and reduced productivity. However, the integration of computer vision technologies and advanced smart harvesting equipment, including automated harvesters and vision-guided picking robots, marks a significant advancement in the automation of crop collection.
The primary objective of harvesting activities involves preserving product quality during the picking process to maximize commercial value. Computer vision solutions perform tasks like automatically harvesting avocados in controlled greenhouse settings or the automated detection of strawberries in open-field conditions.
Automated Weeding
In agronomy, weeds represent unwanted vegetation because they compete with cultivated plants for moisture, nutrients, and minerals present in the soil. Applying herbicides to the exact locations where weeds grow significantly lowers the risk of exposing crops, humans, animals, and water supplies to harmful chemicals.
The smart identification and targeted elimination of weeds are essential for the advancement of modern agriculture. A neural network-based computer vision system recognizes potato crops and distinguishes multiple different weed species for herbicide application.
Crop Analysis
The output and quality of key crops like rice and wheat play a vital role in maintaining food supply stability. In the past, monitoring crop development mainly depended on subjective assessments by humans, which caused delays and proved imprecise. Computer vision solutions offer continuous, non-invasive observation of plant growth and their reaction to nutrient levels.
Using computer vision technology, real-time tracking of crop development can identify minute variations caused by nutrient deficiencies much earlier than traditional methods, offering a trustworthy, precise foundation for making timely adjustments.
Computer vision systems also evaluate plant growth parameters and identify developmental stages.
Crop Yield Tallying
By applying computer vision technology, companies enjoy automated soil monitoring, ripeness assessment, and crop yield prediction. Current technologies integrate with spectral imaging and deep learning models for extra power and control.
Computer vision offers high accuracy, low operational cost, easy portability, seamless integration, and expandability, supporting informed management decisions. One example involves estimating apple production by detecting and counting fruits through computer vision-based systems.
Harvest output from cotton plantations can be forecasted by analyzing imagery captured by unmanned aerial vehicles (UAVs).
Disease Detection
Automated, precise assessment of disease intensity proves crucial for maintaining food safety, effective disease control, and forecasting crop losses. Deep learning eliminates the need for manual feature extraction and threshold-dependent image segmentation.
Image-based, automatic estimation of plant disease severity uses deep convolutional neural network applications to detect fungal infections in wheat and apple black rot.
Drone Monitoring
Up-to-the-minute farmland data and an accurate interpretation of that information remain fundamental elements of precision farming. Unmanned aerial vehicles (UAVs) support the collection of high-resolution agricultural data at a low expense and with swift deployment.
Through ongoing crop surveillance, UAV systems with imaging sensors deliver in-depth insights into agricultural economics and crop health. UAV-based remote sensing leads to higher agricultural yields and reduces farming expenses.
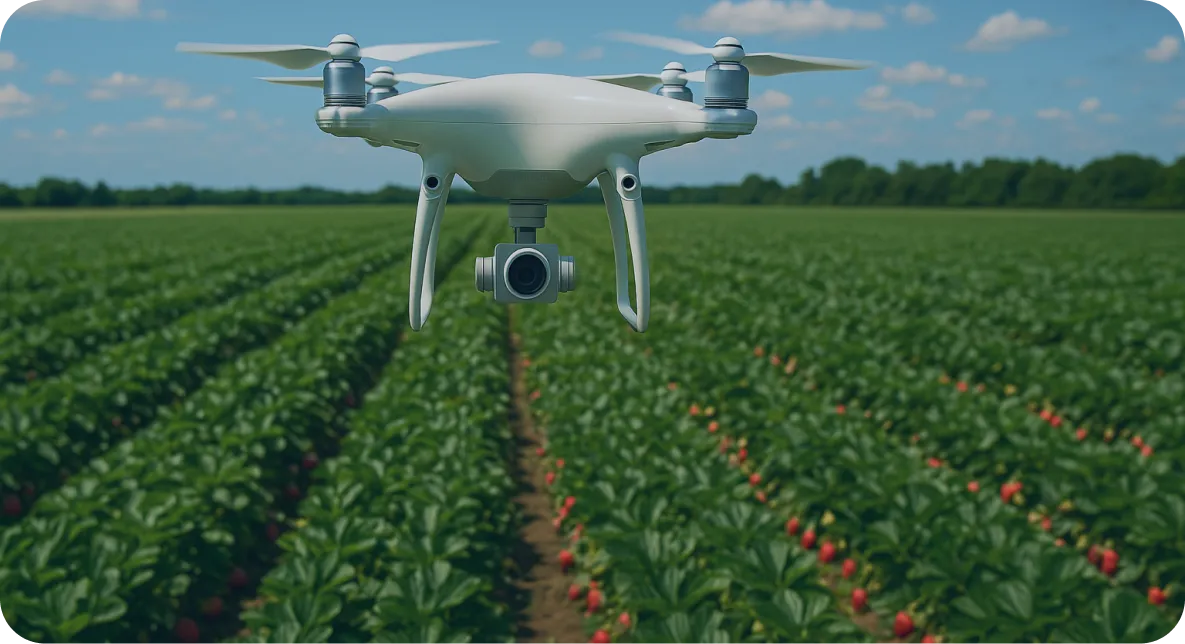
Flowering Alerts
The heading time for wheat remains one of the crop’s most critical growth indicators. An automated computer vision monitoring system identifies the wheat heading stage as soon as it starts.
Computer vision techniques offer benefits such as affordability, minimal error margin, high performance, and strong reliability, supporting real-time, continuous analysis.
Irrigation Management
Soil management practices that implement the latest technology to improve soil fertility through tillage, nutrient application, and watering significantly influence contemporary farming practices. By gathering relevant data on the development of horticultural plants through visual imagery, computer vision can precisely assess soil moisture balance for accurate irrigation scheduling.
Computer vision solutions offer valuable insights into water balance for irrigation planning. An AI vision system analyzes multispectral images captured by unmanned aerial vehicles and extracts the vegetation index (VI) to deliver data-backed support for irrigation decision-making.
Livestock Monitoring
Livestock surveillance is a crucial component of intelligent agriculture. ML algorithms use video feeds to observe the well-being of swine, cattle, and poultry. Intelligent vision systems evaluate animal behavior, health conditions, and overall welfare, improving output levels and economic gains.
Pest Detection
Fast, precise detection and enumeration of airborne insects are essential for pest management. However, conventional manual methods of identifying and counting flying insects prove time-consuming and labor-intensive. AI vision unlocks the recognition and quantification of flying pests with object detection and classification models such as You Only Look Once (YOLO).
Plantation Surveillance
In smart farming, image analysis using aerial drone photographs assists with the remote observation of rapeseed oil farms. By taking geospatial orthographic images, one can determine which sections of the plantation area appear the most productive and suitable for crop cultivation.
Computer vision also detects zones with lower fertility based on plant growth performance. OpenCV remains a widely used library for handling such image-processing operations.
Quality Control
The grade of agricultural produce influences its market value and buyer satisfaction. In contrast to manual evaluations, computer vision offers a faster method for conducting external quality assessments.
Artificial intelligence vision systems offer high adaptability and consistent performance at a comparatively lower expense while maintaining strong accuracy. For instance, platforms utilizing ML and computer vision technologies rapidly detect key lime defects and perform non-invasive quality analysis of yams.
Computer Vision in Healthcare
Medical imaging information has proven to be one of the most valuable and data-rich resources available.
In the absence of suitable technology, physicians must dedicate hours to manually reviewing patient records and handling clerical tasks.
In response, the healthcare sector has become one of the quickest industries to embrace new automation technologies, including computer vision apps.
Let’s examine some of the most widely used computer vision applications in medical care.
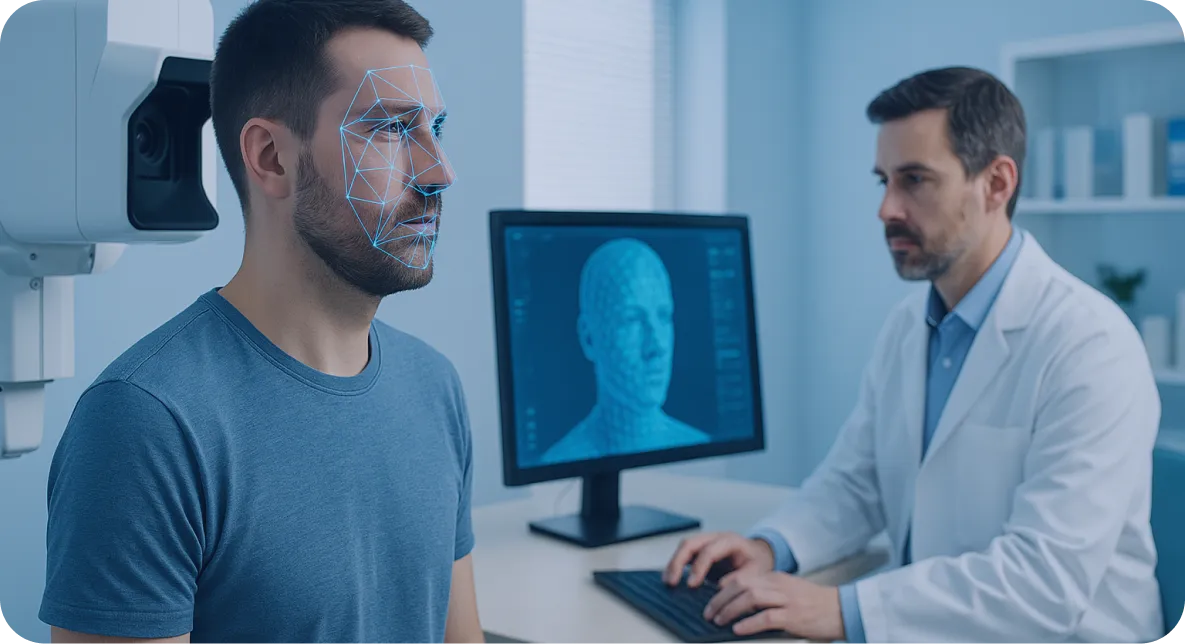
Blood Loss Measurement
Postpartum bleeding remains one of the leading causes of maternal death during childbirth. In the past, doctors could only estimate the volume of blood a patient had lost during labor.
With the help of computer vision, physicians can now quantify blood loss by using an AI iPad system that analyzes images of surgical sponges and suction containers.
Findings revealed that in most cases, physicians tend to overcalculate the amount of blood lost during delivery.
As a result, computer vision enabled more precise blood loss assessments, allowing healthcare providers to deliver faster, more effective treatment.
Cell Classification
Machine learning for healthcare applications distinguishes T-lymphocytes from colon cancer epithelial cells with remarkable precision. Technologists anticipate that ML will significantly speed up the detection of colorectal cancer at a minimal cost.
COVID-19 Diagnosis
Computer vision applied to coronavirus management during the pandemic. Numerous deep learning-based vision models for COVID-19 detection use chest X-ray imaging. Darwin AI created COVID-Net, one of the most widely used frameworks for identifying COVID-19 infections through digital chest radiographs.
CT Scan and MRI Analysis
Computer vision sees extensive use in the interpretation of CT and magnetic resonance imaging (MRI) scans. From analyzing radiological images with human-level accuracy to enhancing the image clarity of MRI scans, computer vision plays a central role in elevating patient care. One example involves ML-powered annotation of the brain and spinal cord.
Employing computer vision techniques to examine CT and MRI imagery assists medical professionals in identifying tumors, internal hemorrhaging, blocked arteries, and other critical medical issues. The automation of this process also improves diagnostic precision because machines can recognize minute details imperceptible to the naked eye.
Digital Pathology
Due to the widespread adoption of whole-slide imaging (WSI) digital scanning devices, computer vision now performs medical image analysis to detect and classify the type of pathology being observed. In digital pathology, doctors and technicians use computer vision:
- Image examination and interpretation
- Thorough analysis of tissue samples
- Matching pathology types with historical cases
- Increasing diagnostic precision for earlier detection
Computer vision digital pathology has boosted the accuracy and speed of diagnoses, allowing physicians to conserve valuable time and make more informed clinical decisions.
Disease Progression Scoring
Computer vision detects disease in severely ill individuals and prioritizes medical intervention for critical patient triage.
Individuals infected with COVID-19 often exhibit accelerated breathing rates. Deep learning algorithms combined with depth-sensing cameras recognize irregular breathing behaviors, supporting a precise, non-invasive, and large-scale assessment of people potentially infected with the COVID-19 virus.
Mask Compliance
Facemask recognition identifies the presence of face coverings and personal protective gear to reduce the transmission of the coronavirus. Computer vision technologies assist with the enforcement of mask usage as a preventive measure to control the spread of COVID-19.
Private enterprises like Lyft have developed computer vision functionalities that integrate facial recognition into their mobile applications, supporting the detection of masks on drivers and riders. These initiatives contributed to making public transit systems more secure during the COVID-19 outbreak.
Mobility Analysis
Pose estimation evaluates patient mobility and supports physicians in identifying neurological and musculoskeletal diseases.
While most human pose estimation methods are designed with adults in mind, this computer vision approach has also been utilized for infant motion monitoring.
By observing and analyzing an infant’s natural movements, healthcare professionals can anticipate neurodevelopmental conditions at an early stage and implement timely interventions. An automated movement assessment system can record infant body motions and detect irregularities far more effectively than a human.
Rehabilitation
Physiotherapy plays a crucial role in the recovery process for stroke patients and individuals with sports injuries. The primary obstacle to rehabilitation is the expense.
At-home exercises using a vision-enabled rehabilitation system work well because they allow patients to engage in movement therapy in a more private, cost-effective manner. In computer-assisted treatment and rehabilitation, human motion analysis helps patients train at home, guides them toward executing movements correctly, and prevents fresh injuries.
Skin Cancer Detection
Although radiology has quickly embraced computer vision, this technology proves equally impactful in dermatology. According to City of Hope, skin cancer remains the most prevalent form of cancer globally. As with other types of cancer, early identification is essential.
Computer vision initiatives improve the detection of skin cancer while easing the burden on healthcare providers. These vision-based solutions can even empower patients with screening capabilities through mobile phone applications.
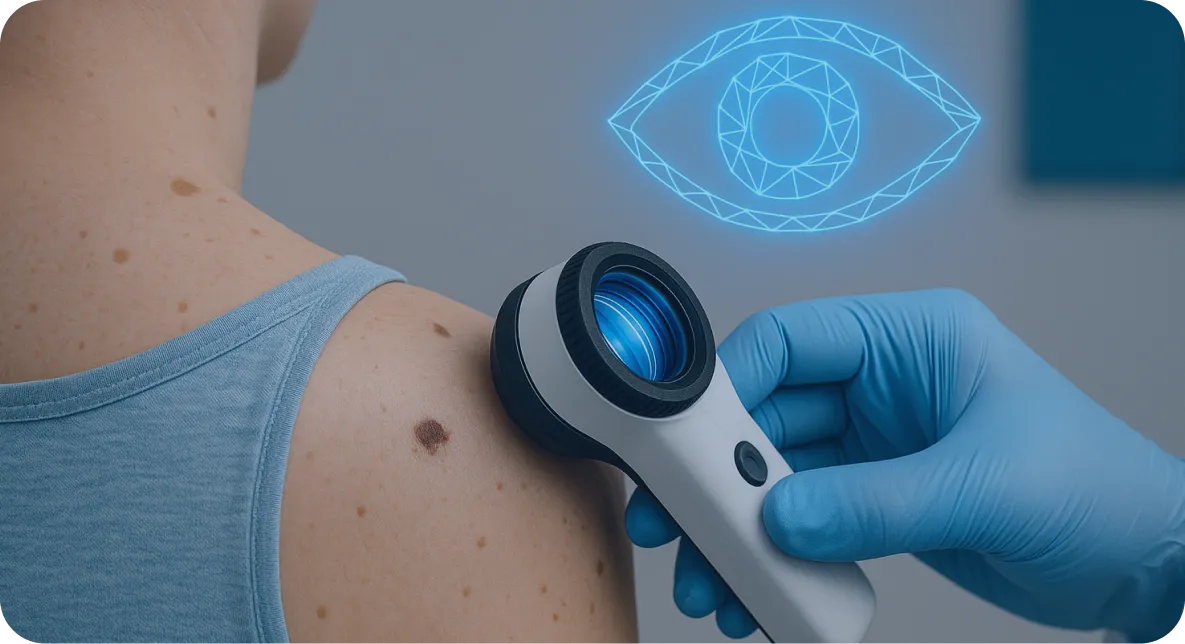
Staff Training
Computer vision applications evaluate the proficiency of advanced students on autonomous learning platforms. For instance, augmented reality (AR) simulated surgical training systems support medical education by giving students the experience they need without risking the health of patients.
Computer vision quality control uses algorithmic techniques to automatically assess the performance of surgical trainees. As a result, educators and students receive valuable feedback to guide and accelerate their skill development.
X-Ray Analysis
In medical X-ray imaging, computer vision sees use in clinical care, scientific research, MRI image reconstruction, and surgical planning.
Although many physicians still depend on manual examination of X-ray scans for diagnosing and treating illnesses, computer vision automates this workflow, increasing speed and precision.
Advanced image recognition models detect patterns in X-ray visuals that prove too subtle for human perception.
Computer Vision in Transportation
The growing needs of the transportation industry have driven several technological advancements, including computer vision.
From self-driving cars to parking space monitoring, the Intelligent Transportation Systems (ITS) have emerged as a vital area for improving the efficiency, performance, and safety of traditional transportation systems.
Let’s explore some of the computer vision use cases in transportation.
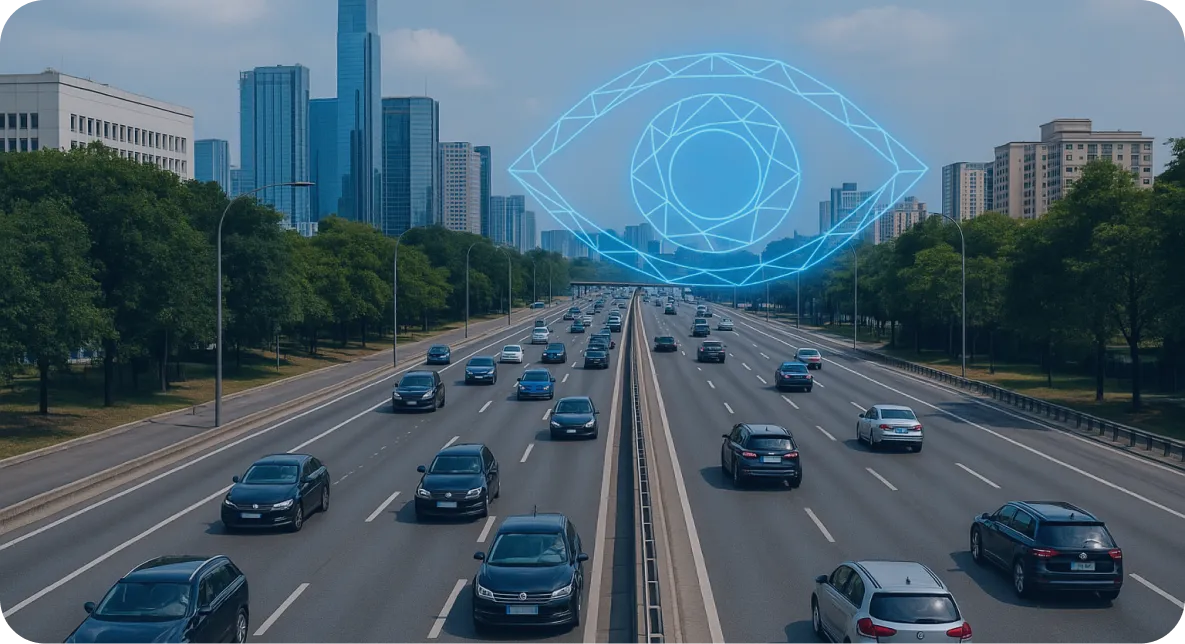
Automated License Plate Recognition (ALPR)
Several contemporary transportation and public security systems depend on the detection and extraction of license plate data from static images and video footage. ALPR has revolutionized the transportation and public safety sectors in recent years.
These number plate identification technologies support modern toll road operations, delivering significant cost reductions through automation and unlocking new functionalities in the industry, including mounted police vehicle license plate scanners.
Autonomous Vehicles
Today, autonomous vehicles are no longer science fiction, but science fact. Thousands of engineers and software developers around the globe actively test and improve the safety and dependability of driverless cars.
Computer vision identifies and categorizes road signs and traffic signals, generates 3D environment models, and performs motion analysis, turning self-driving technology into a tangible reality.
Autonomous vehicles gather data about their environment using cameras and sensors, process the input, and react accordingly.
Researchers involved in Advanced Driver-Assistance Systems (ADAS) integrate computer vision methods like pattern recognition, feature identification, object detection, and 3D perception to create real-time algorithms that support and improve driving functions.
Collision Avoidance
Vehicle recognition and lane identification remain essential components of most autonomous driving and advanced driver assistance systems. In recent years, deep neural networks have developed deep learning techniques with applications in self-driving collision prevention systems.
Congestion Analysis
Traffic movement analysis has been thoroughly researched for intelligent transportation systems using invasive techniques like tags and embedded road sensors, as well as non-intrusive approaches like video surveillance.
With the advancement of computer vision and artificial intelligence, video analysis now applies to traffic cameras, significantly impacting ITS infrastructure and smart urban environments. Government employees can now monitor traffic flow with vision-based technologies, measuring parameters essential for traffic management.
Driver Alertness Detection
Distracted driving detection identifies behaviors like daydreaming, mobile phone usage, and gazing outside the vehicle to prevent a significant portion of traffic-related deaths. Artificial intelligence understands driver actions and develops strategies to reduce road accidents.
Traffic monitoring systems capture violations within the vehicle cabin, such as seat belt non-compliance, using deep learning-based detection models in roadside surveillance. In-vehicle driver observation systems emphasize visual sensing, behavioral analysis, and real-time feedback.
Computer vision evaluates driver conduct directly through inward-facing cameras and indirectly via external sensors and dashcams. Driver-facing video analysis techniques use algorithms to detect faces and eyes, track gaze direction, estimate head position, and monitor facial expressions.
Facial recognition algorithms have successfully distinguished between alert and distracted facial states. Deep learning models identify differences between focused and unfocused eyes, along with indicators of impaired driving.
Various vision-driven applications support real-time classification of distracted driver postures, utilizing a combination of deep learning architectures like recurrent neural networks (RNNs) and convolutional neural networks (CNNs) to enable immediate detection of driver inattention.
Infrastructure Condition Updates
To guarantee the safety and operational integrity of civil infrastructure, the government must perform visual evaluations and analyze its physical and functional state. Computer vision-enabled systems for infrastructure inspection and surveillance automatically transform images and video streams into meaningful, actionable insights.
Intelligent inspection tools recognize structural elements, classify visible deterioration, and identify deviations from baseline imagery. These monitoring solutions support static assessments of strain and movement, as well as dynamic tracking of displacement for purposes such as modal analysis.
Intelligent Toll Booths
Modern toll collection systems no longer require motorists to halt and pay with exact cash. They leverage computer vision to automatically process payments, identify rule violators, and monitor traffic patterns in real time.
Smart tolling technologies categorize vehicles by type to charge the correct toll fee. License plate recognition solutions employ OCR to extract plate numbers from images and video feeds of passing vehicles. The system then cross-references those numbers with a vehicle registration database, matching the plate with a driver’s toll account or determining the address for the billing notice.
Parking Occupancy Monitoring
Visual parking area monitoring achieves accurate detection of occupied and available spaces. Particularly in smart urban environments, computer vision technologies enable decentralized, highly efficient methods for detecting parking space occupancy using deep CNNs.
Video parking control systems use stereoscopic, 3D imaging and thermal cameras. The benefit of camera-based parking space recognition lies in its scalability for widespread deployment, low maintenance costs, and simple installation, especially when repurposing existing surveillance cameras.
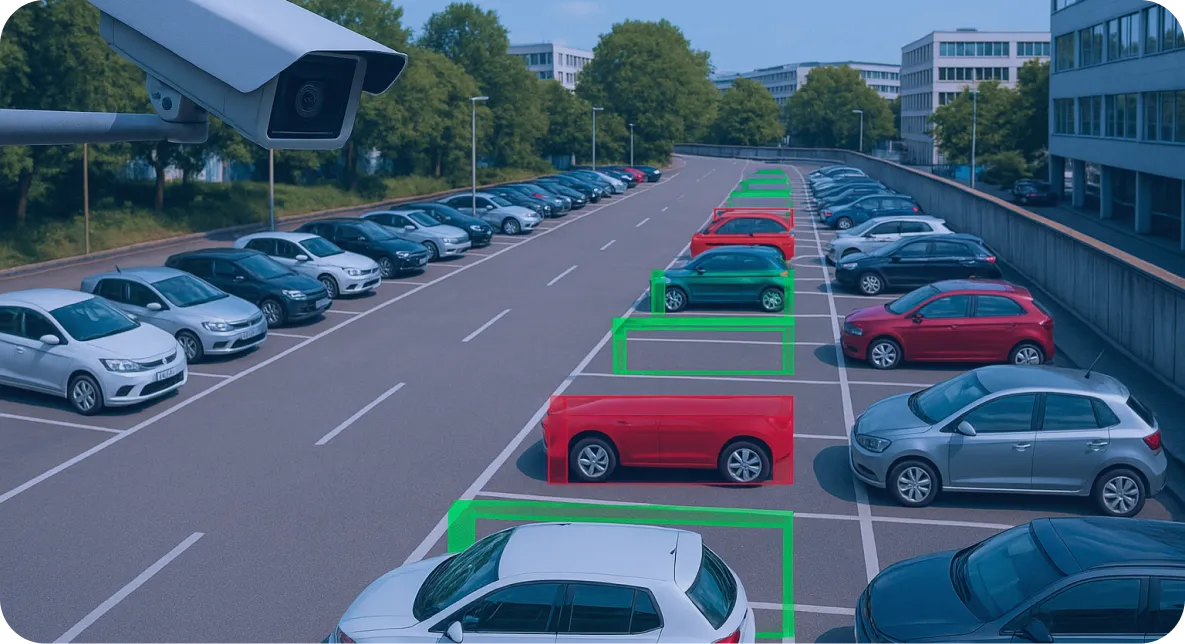
Pedestrian Tracking
Identifying pedestrians is essential for ITS. Computer vision applications include everything from autonomous vehicles to infrastructure monitoring, traffic control, public transit safety and performance, and law enforcement activities.
Pedestrian recognition uses several sensing technologies, including traditional CCTV, IP surveillance cameras, thermal sensors, near-infrared imaging tools, and onboard RGB cameras. Human detection algorithms use infrared heat patterns, body shape descriptors, gradient-based attributes, ML models, and motion-tracking techniques.
Pedestrian identification powered by deep convolutional neural networks has achieved notable advances, including the accurate detection of partially obscured individuals.
Road Condition Updates
Computer vision-enabled defect identification and structural condition evaluation monitor the state of concrete and asphalt civil infrastructure. Pavement surface evaluation delivers insights that support cost-efficient, uniform decision-making for the maintenance and planning of roadway systems.
Governments conduct pavement deterioration surveys using advanced data-gathering vehicles and on-foot visual inspections. Deep learning can generate an asphalt pavement condition index, offering a systematic, affordable, effective, and secure solution for automated pavement damage detection.
Traffic Sign Recognition
Computer vision applications identify and interpret traffic signs. Visual processing methods extract traffic signs from roadway environments through image segmentation, applying deep learning to detect, categorize, and classify traffic signage.
Traffic Violation Alerts
Law enforcement bodies and local governments are expanding the use of camera-equipped roadway surveillance systems to curb hazardous driving practices. One of the most crucial applications involves the identification of stationary vehicles in high-risk zones.
Governments continue to adopt computer vision methods in intelligent urban environments to automate the detection of traffic violations, such as speeding, ignoring traffic signals or stop signs, driving in the wrong direction, and executing illegal turns.
Vehicle Re-Identification
With advancements in pedestrian re-identification, intelligent transportation and monitoring systems aim to apply similar strategies to vehicles through vision-based vehicle re-identification. Traditional methods for assigning a distinct vehicle identity are often invasive, relying on onboard tags, mobile devices, or GPS tracking.
In controlled environments, like toll stations, automatic number plate recognition (ANPR) is the most effective solution for precise vehicle identification. However, drivers can alter or falsify license plates, and ALPR systems can not capture notable visual traits of a vehicle, such as scratches, dents, and other markings.
Non-invasive approaches, like image-based vehicle recognition, show potential and enjoy growing interest, yet remain underdeveloped for widespread real-world deployment. Most current computer vision vehicle re-identification methods rely on external characteristics, including color, surface patterns, and overall form.
At present, recognizing subtle and distinguishing details, like vehicle brand or model year, remains a significant, unresolved challenge.
Vehicle Tracking
Computer vision applications for automated vehicle categorization have a deep history. Technologies enabling automatic vehicle classification for the purpose of traffic counting have gradually progressed over the years. Deep learning techniques now allow for the development of large-scale traffic monitoring systems using standard, low-cost surveillance cameras.
With the increasing availability of budget-friendly sensors such as CCTV systems, LiDAR, and thermal cameras, authorities can detect, monitor, and classify vehicles in multiple lanes simultaneously. The precision of vehicle identification increases by fusing various sensors, including thermal imaging, LiDAR data, and RGB video feeds from commonly used IP or security cameras.
Some companies have adopted deep-learning computer vision for construction vehicle recognition to support safety oversight, efficiency evaluation, and strategic management.
Computer Vision in Financial Services
Safety and fraud prevention are of utmost importance in the finance and banking sectors, and computer vision offers a wide array of solutions. It increases the security of transactions, accelerates insurance claim handling, and improves financial strategy development.

Augmented Reality Financial Planning
Managing finances can become complicated, but augmented reality-based computer vision makes the process more engaging and user-friendly. AR tools permit individuals to visualize live financial metrics, presenting details like spending patterns, investment outcomes, and retirement fund forecasts in an interactive 3D layout. By embedding AR capabilities into banking and wealth advisory platforms, institutions can deliver a more customized, immersive experience for financial planning.
Automated Damage Assessment
Insurance providers have historically depended on manual evaluations and paperwork-intensive procedures to assess losses resulting from accidents or natural disasters. Computer vision now allows insurers to examine photos of damaged vehicles, properties, and other assets to swiftly determine the severity of the damage and estimate repair expenses. This AI-powered method accelerates the claims-handling process, decreases the necessity for on-site assessments, and reduces human mistakes. By automating damage evaluation, insurers offer quicker reimbursements to policyholders while detecting fraudulent claims more effectively.
Biometric ATM Withdrawals
Conventional ATM operations depend on PIN codes and physical bank cards, which get misplaced, stolen, or compromised. With the integration of computer vision, ATMs can verify user identity through facial recognition, permitting customers to withdraw money simply by facing the camera on the machine. This technology boosts security by ensuring that only the legitimate account owner can access their funds, minimizing the risk of card skimming, PIN code theft, and unauthorized usage. Biometric authentication also accelerates the cash withdrawal process, making transactions more efficient and reducing queue times at ATMs.
Computer Vision in Sports
Sports fans already bear witness to the impact of computer vision and AI in sports. Ball-tracking, computer vision-assisted reviews, and augmented replays represent just a few of the most common use cases. Let’s examine how sports leagues have implemented the latest computer vision products in games and on the practice field.

Augmented Coaching
Computer vision-powered sports video analysis optimizes resource utilization and shortens feedback delivery durations for time-sensitive activities. Coaches and athletes engaged in time-consuming notational analysis, such as post-race swim evaluations, can receive fast, objective feedback before the following performance.
Self-guided training systems for athletic activities have recently become a growing area of computer vision research. Although independent practice remains crucial in sports, a trainee’s development will stall without guidance from a coach.
For instance, a yoga self-practice application can guide users in executing yoga poses properly, correcting improper alignment and reducing the risk of injury. Vision-based training platforms can also offer real-time guidance on how to adjust body positioning.
Augmented Judges’ Scoring
Deep learning techniques evaluate the quality of athletic movements supporting automated scoring systems for sports such as diving, boxing, figure skating, and gymnastics.
For instance, a diving assessment tool functions by calculating a quality score based on an athlete’s dive execution. It examines the positioning of the feet to determine if:
- The feet remain together
- The toes are pointed
- The body maintains a straight posture throughout the entire dive sequence.
Ball Tracking
Real-time object tracking identifies and records movement patterns of balls and pucks. Ball trajectory information assesses athlete shot performance and informs the study of game tactics. Ball motion tracking involves locating and continuously monitoring the ball across a series of video frames.
Ball movement analysis appears especially valuable in large-field sports because it supports faster interpretation and evaluation of gameplay and strategic decisions.
Biomechanics Tracking
AI-powered vision systems identify patterns in human body movements and postures across multiple video frames and live streams. For instance, human pose estimation in real-world footage of swimmers uses fixed-position cameras to capture views from above and below the waterline.
Coaches can use these video recordings to quantitatively evaluate athletic performance without manual labeling body parts in each frame. CNNs automatically derive the necessary pose data and identify swimming techniques performed by the athletes.
Event Marking
Computer vision identifies complex occurrences from unstructured video content, such as goals, close attempts, or other non-scoring activities. This technology is valuable for real-time event recognition during sports broadcasts and can be applied across a broad spectrum of field-based sports.
Goal Line Technology
Camera systems can verify the legitimacy of a goal, assisting referees in their decision-making process. Unlike sensor-based approaches, this AI vision method remains unintrusive and does not necessitate modification to standard football equipment.
These goal-line technology solutions rely on high-speed imaging devices that use captured frames to triangulate the ball’s location. A ball recognition algorithm evaluates potential ball regions to identify the ball’s distinctive pattern.
Highlight Reel Generation
Creating sports highlight reels represents a time-consuming task that demands a certain level of expertise, especially for sports with complicated rules and lengthy gameplay durations. Automated baseball highlight creation leverages event-based, excitement-level indicators to identify and extract key match moments.
Another use case involves the automatic compilation of tennis highlights using multimodal excitement cues combined with computer vision techniques.
Motion Capture
Cameras utilize pose estimation to monitor human skeletal movement without relying on conventional optical markers or specialized recording equipment. This capability proves crucial in sports motion capture during games, where athletes cannot perform while wearing cumbersome tracking suits or extra hardware.
Opponent Analysis
Expert sports analysts frequently conduct evaluations to obtain strategic, tactical understanding of individual player actions and team dynamics. However, manual review of footage proves labor-intensive, requiring analysts to remember and label video segments.
Computer vision methods extract movement trajectory data from video recordings and utilize motion analysis algorithms to examine:
- Field zones
- Team arrangements
- Game incidents
- Athlete movements
Performance Analysis
Automated identification and classification of sport-specific actions address the drawbacks linked to manual performance evaluation techniques, such as bias, lack of measurable metrics, and limited repeatability. Computer vision data comes from inputs like body-mounted sensors and wearable devices. Common applications include swimming technique evaluation, golf swing assessment, running gait analysis, snowboarding performance monitoring, and the detection and analysis of pitching actions.
Swing Recognition
Computer vision identifies and categorizes sports strokes in golf, tennis, baseball, and cricket. Motion recognition and action classification involve interpreting movements and generating labeled predictions for the observed action, such as backhand and forehand strokes.
The purpose of stroke detection involves evaluating matches and improving athletic performance more effectively.
Teamwork Tracking
By applying computer vision algorithms, computers can estimate the human posture and body motion of several team athletes from single-camera recordings and multi-camera footage in sports video datasets. The 2D or 3D pose estimation of multiple players proves valuable for performance evaluation, motion tracking, and emerging use cases in sports broadcasting and immersive fan experiences.
Get the Latest Computer Vision Solutions From AiFA Labs
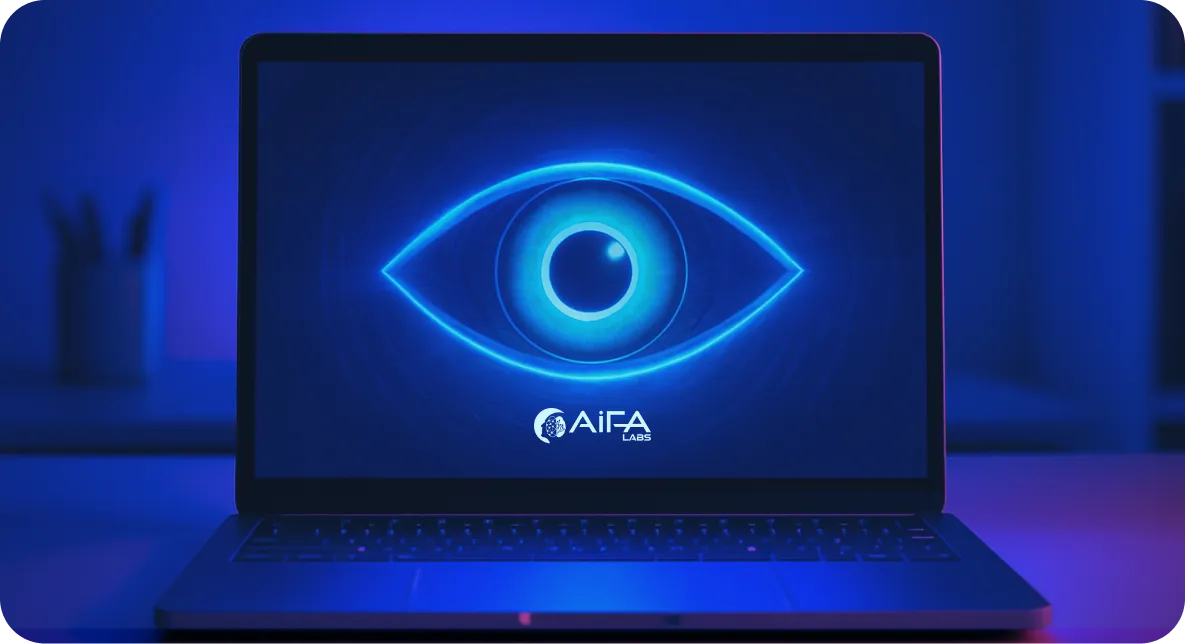
Now that you understand the most popular computer vision applications within your industry, discover how object detection, image processing, and automated video analysis can optimize your business. Our Edge Vision AI Platform revolutionizes operations for businesses in any sector. Request a free demonstration online or call AiFA Labs at (469) 864-6370 today!