Discover how to promote AI safety through artificial intelligence governance. The use of AI systems has far-reaching societal implications and presents some risks to data security. Responsible AI development requires companies to institute proper governance in all AI models. Below, we explore what AI governance is and why it is necessary.

What Is AI Governance?
AI governance is a compendium of artificial intelligence frameworks, policies, and best practices that comprise guardrails for the development and usage of AI systems. It reduces the risks of AI bias and increases the benefits of AI technologies. But is an artificial intelligence governance framework necessary? Let’s find out!
Why Is AI Governance Necessary?
AI governance is necessary to align AI technology with a company’s business objectives, strategies, and values while complying with the latest federal and state legislation, administrative rules, and other legal regulations. The main purposes behind governance practices relate to public trust, regulatory compliance, risk mitigation, societal considerations, and transparency.
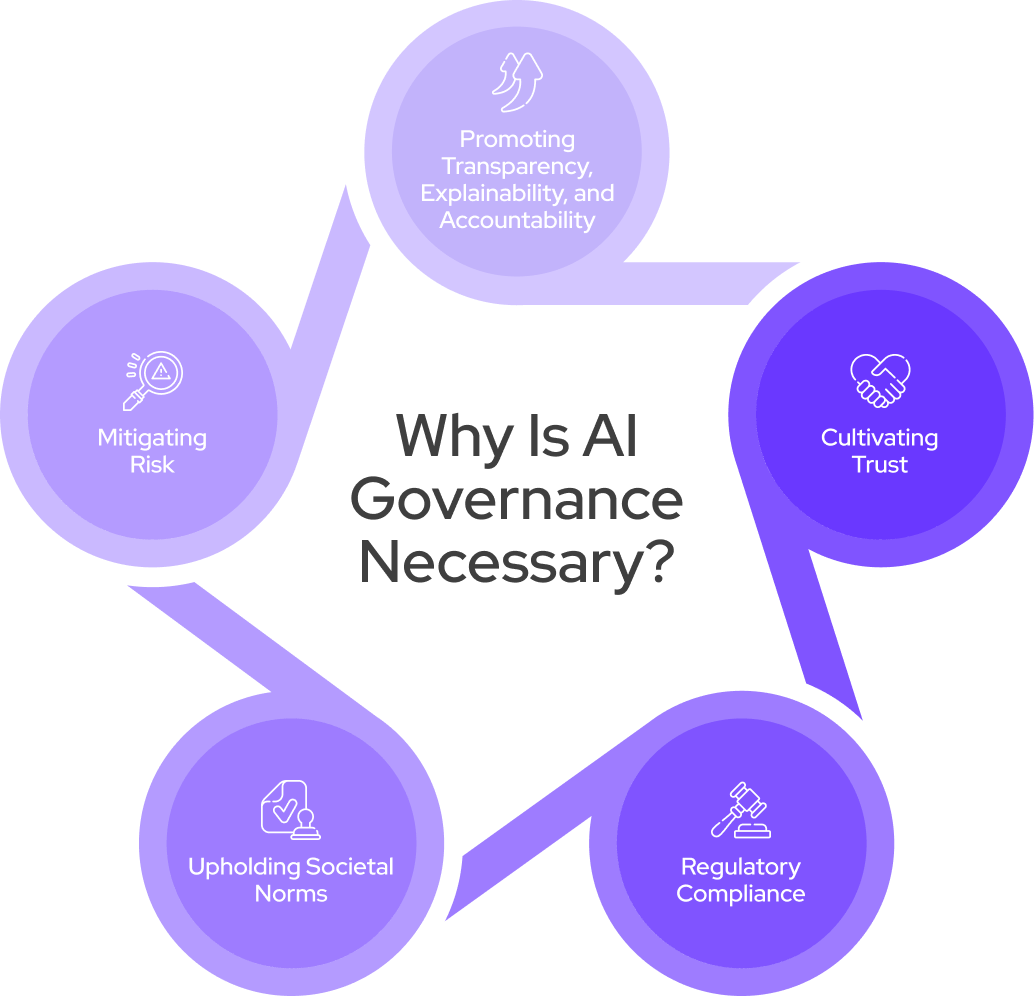
Cultivating Trust
By their nature, AI systems lack transparency in some areas. For example, the sheer volume of information contained with a generative AI system rules out manual, human review. It also highlights the need for strict governance of sensitive data. Generative AI governance standards should force AI businesses to hand over details about AI algorithms and data sources. Doing so would cultivate trust among diverse stakeholders, employees, and customers.
Regulatory Compliance
Global AI governance policies aim to guide the development of artificial intelligence (AI) in compliance with the law. Governing bodies around the world are drafting, enacting, and promulgating new laws to regulate both public and private sectors, like the EU AI Act. Effective AI laws will promote AI ethics that protect users’ data and reduce potential harm. Organizations like the AI Governance Alliance of the World Economic Forum assist governments by drafting proposed AI legislation.
Mitigating Risk
The latest AI systems contain some governance components to mitigate risk through AI governance best practices. Some AI-related risks include broken trust, overreliance on GenAI models, and bias in decision-making processes. AI governance addresses all of these issues through ongoing monitoring, human intervention, and the tracking of governance metrics.

Upholding Societal Norms
The current discussions surrounding AI ethics focus primarily on the need to ensure AI systems make fair and unbiased decisions. Trustworthy AI will reflect careful consideration from AI research and development teams who focus on risk assessment, ongoing monitoring, and risk management. AI model development should center around ethical standards that promote fairness, transparency, and accountability.
Promoting Transparency, Explainability, and Accountability
AI governance aims to promote transparency, explainability, and accountability as AI systems make increasingly important decisions. It helps us understand how AI reaches its conclusions and holds systems accountable for unfair outcomes. Governance also ensures responsible use as companies adapt to the AI era. Governance remains key to maximizing AI’s benefits while addressing risks.
The Keys to Responsible AI Governance
As artificial intelligence continues to promote technological advancement, the need for robust, responsible AI governance has never been more critical. Five fundamental pillars that form the foundation of ethical AI development and deployment: privacy, security, fairness, transparency, and accountability. By understanding and implementing these key principles, organizations can mitigate risks and harness the full potential of AI. Find a discussion on the keys to responsible AI governance below.

Privacy
Data privacy considerations remain a critical factor in the regulatory frameworks governing artificial intelligence systems because AI technologies frequently aggregate and process sensitive personally identifiable information (PII). Companies must formulate and implement comprehensive protocols for data stewardship to safeguard privacy rights and other human rights in accordance with relevant legislation and ethical standards.
Security
Cybersecurity is a critical component in AI governance frameworks, with particular emphasis on:
- Data confidentiality
- Data integrity
- Mitigation of cyberattacks
- Identification of system vulnerabilities
Implementation of comprehensive security protocols and robust defense mechanisms is essential to fortify AI systems and their associated data assets against malicious threat actors. The development of AI governance models remains inherently heterogeneous, with diverse jurisdictions and entities prioritizing different objectives and risk factors, leading to disparate regulatory landscapes and compliance requirements.
For initial guidance, practitioners may refer to standardized AI risk assessment methodologies, such as the National Institute of Standards and Technology’s Artificial Intelligence Risk Management Framework (NIST AI RMF), which provides a structured approach to identifying, evaluating, and mitigating AI-specific risks.
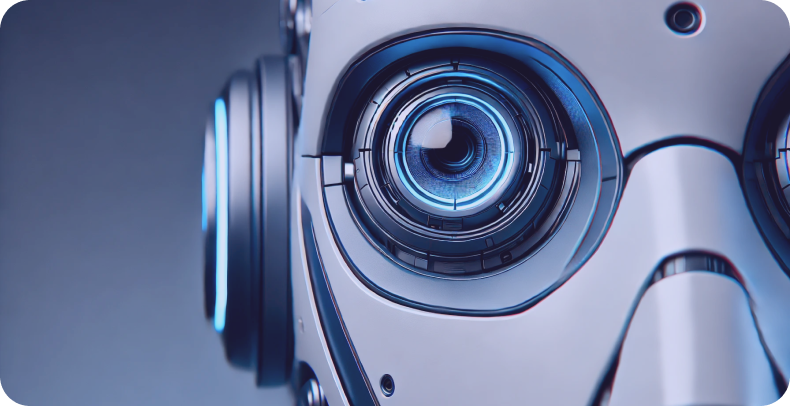
Fairness
Equitable AI governance policies mandate the elimination of discriminatory outcomes from AI systems. Engineers implement various strategies to protect human rights through AI fairness, including:
- Bias detection algorithms to scrutinize training datasets
- Stratified sampling techniques to maintain representational balance
- Fairness metrics to quantify model performance
- Decision boundary optimization algorithms recalibration to yield equitable outcomes
Continuous monitoring protocols track fairness metrics as systems analyze novel data, encouraging equity in algorithmic decision-making.
Transparency
Algorithmic transparency requires exposing the internal mechanisms of AI systems to scrutiny by diverse stakeholders impacted by their outputs. Developers implement multiple strategies to achieve transparency, including:
- Comprehensive system architecture documentation to delineate model components and data flows
- Open-source codebases to enable peer review and community-driven improvements
- Rigorous testing protocols to validate model performance across diverse scenarios
- Formal verification methods to prove algorithmic properties and invariants
These practices elucidate the decision-making processes of AI systems, facilitating informed discourse on their societal implications.
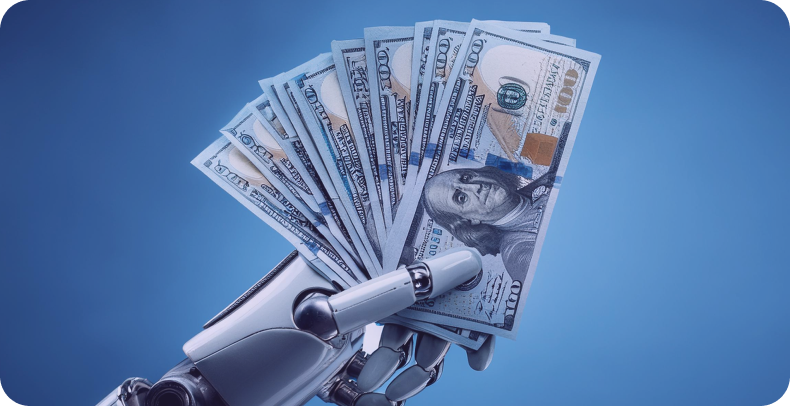
Accountability
Accountability, a fundamental tenet of AI governance, requires diverse stakeholders to assume direct responsibility for AI system development, deployment, and outcomes. Regulatory bodies must implement and enforce compliance frameworks to hold companies accountable.
Specifically, the principle of accountability requires that:
- Developers implement audit trails and logging mechanisms to track decision processes
- Organizations establish clear chains of responsibility for AI-powered outcomes
- Regulatory agencies enact penalties and remediation protocols for non-compliance or adverse impacts
- Ethics boards conduct regular reviews of AI systems’ societal effects
- Legal frameworks evolve to address liability issues related to AI
These measures create a robust accountability ecosystem, ensuring stakeholders proactively manage AI risks and address potential negative externalities.
Best Practices for AI Governance
As artificial intelligence permeates most areas of society, responsible governance becomes paramount. Five critical aspects of AI governance help develop and deploy AI systems that are technologically advanced, ethically sound, transparent, and aligned with societal values. Let’s explore some of the best practices for AI governance.

Assess AI’s Impact on Society
AI governance frameworks require strict data privacy protocols and bias mitigation strategies. Robust sampling methodologies, diversified development teams, and curated, high-quality training datasets can minimize algorithmic discrimination. Risk management strategies, including fairness metrics and decision boundary optimization, remain essential for responsible AI model deployment. Ongoing monitoring and recalibration processes promote ethical AI standards.
Implement Internal AI Governance
AI governance calls for sophisticated internal frameworks. Cross-functional teams composed of AI specialists, executives, and stakeholders can formulate and implement comprehensive policies governing AI utilization.
These frameworks define use cases, delineate roles, enforce accountability, and facilitate outcome assessments. They establish protocols for model development, deployment, and monitoring to maintain alignment with organizational objectives and current regulations.

Manage AI Systems
Companies must implement robust AI model lifecycle management protocols to mitigate performance degradation. Automatic monitoring systems detect model drift through statistical analysis of input distributions and output metrics. Automated retraining pipelines leverage fresh data to maintain model accuracy.
Rigorous A/B testing frameworks evaluate updated models against baseline performance. These processes ensure AI models maintain optimal predictive power and adhere to defined fairness criteria throughout their operational lifespans.
Engage Stakeholders
Organizations should set up comprehensive stakeholder communication standards to promote transparency in AI development and deployment. These protocols should explicate methodologies for disseminating technical information, use cases, and impact assessments to all stakeholder groups, including employees, end-users, investors, and community members.
Formalized engagement policies facilitate the elucidation of AI architectures, decision-making processes, and potential externalities. This proactive approach fosters trust, mitigates reputational risks, and aligns AI initiatives with stakeholder expectations and societal values.

Focus on Data Governance
Businesses can build rigorous data governance frameworks to safeguard sensitive consumer information. These frameworks may have robust encryption protocols, access control mechanisms, and data anonymization techniques.
By enforcing stringent data quality standards and compliance with privacy regulations, organizations mitigate risks of data breaches and misuse. An AI governance framework should address data lineage, provenance tracking, and ethical use guidelines to maintain the integrity of AI outcomes while preserving consumer privacy for the entire data science lifecycle.
Governing AI Technologies | Cerebro by AiFA Labs
Build the perfect AI governance framework with the Cerebro GenAI Governance Portal. Our cutting-edge AI tools keep companies up to date and compliant with the latest AI regulations. Book a free demonstration online or call us today at (469) 864-6370 to start fostering innovation at your company.